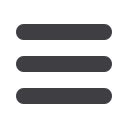
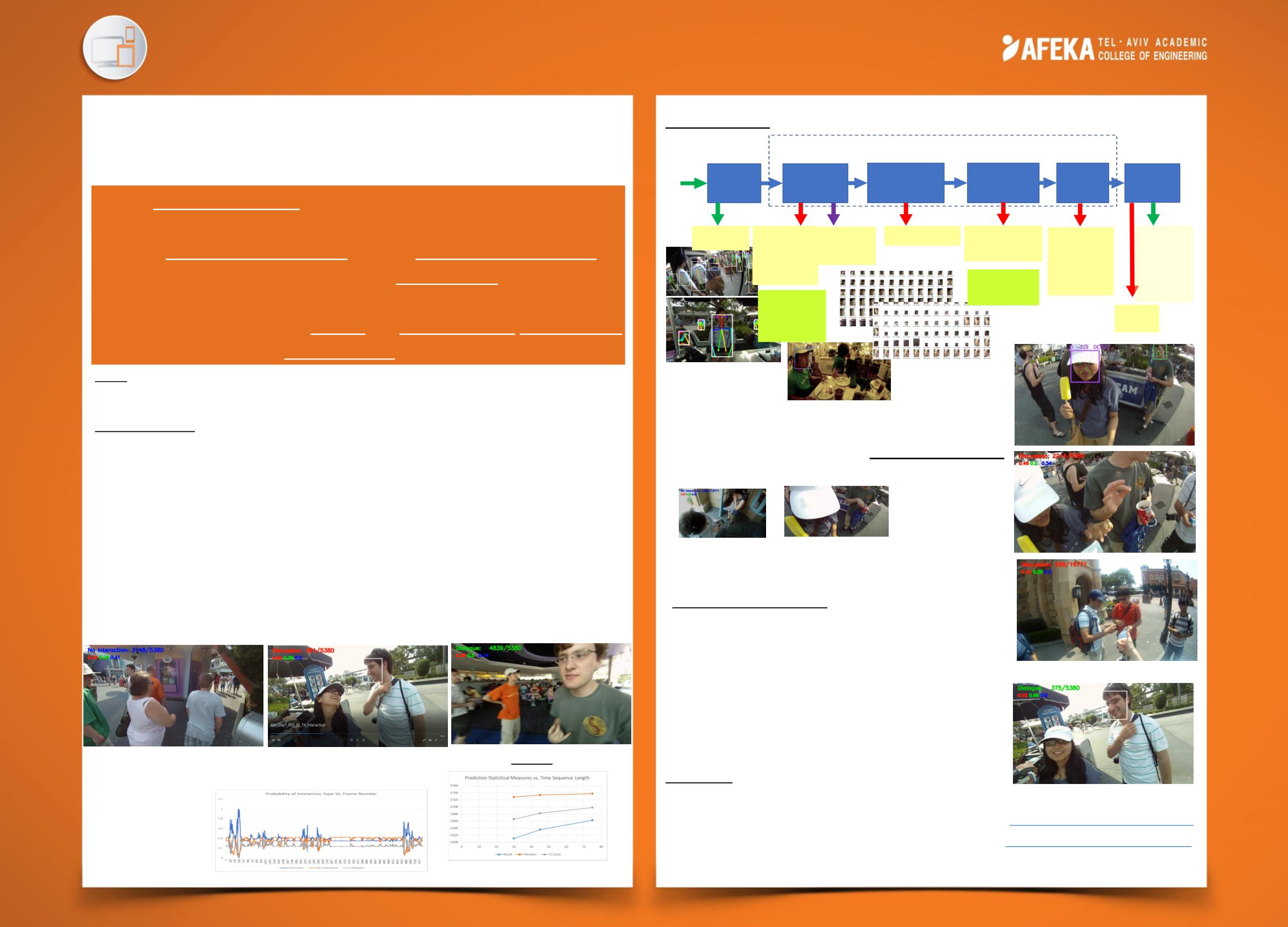
Detection of Interpersonal Interactions in Egocentric
Video using Deep Learning
Goals
Detect Interpersonal Interactions in Egocentric Video; Create summary video of these
“important” moments where interpersonal interactions occurred.
Processing stages
•
Data preprocessing
:lens distortion correction and data separation
•
Feature extraction
:generate features from each frame using:
•
face detection, face tracking (Bounding box, facial landmarks, Temp ID)
•
face recognition (ID unification)
•
Feature fusion
: sort features; select the “most interesting” persons per frame,
prepare temporal sequence of feature vectors
•
Sequence classification
:temporal classification for: multiple activities: “Dialogue”,
“Discussion”, “No Interaction”
•
Video summarization: summary video that contains only scenes with interactions
•
All interpersonal interactions
•
All appearances of a specific Person of Interest (
POI
) interactions
•
All No-interactions.
•
Results
Over 94% true detection of interaction labels
Barak Katz
Supervisor: Dr. Alexander Apartsin
Software Engineering
The solution:
References:
[1]. del Molino, Ana Garcia, Cheston Tan, Joo-Hwee Lim, and Ah-Hwee Tan. "Summarization of Egocentric Videos: A Comprehensive Survey." IEEE
TRANSACTIONS ON HUMAN-MACHINE SYSTEMS 47, no. 1 (2017): 65-76, Feb. 2017, doi: 10.1109/THMS.2016.2623480..
[2]. Ghafoor, Humaira A., Ali Javed, Aun Irtaza, Hassan Dawood, Hussain Dawood, and Ameen Banjar. "Egocentric Video Summarization Based on People
Interaction Using Deep Learning." Mathematical Problems in Engineering 2018 (2018).
https://www.hindawi.com/journals/mpe/2018/7586417/[3]. Bano, Sophia, Tamas Suveges, Jianguo Zhang, and Stephen J. Mckenna. "Multimodal Egocentric Analysis of Focused Interactions."
IEEE Access
6
(2018): 37493-37505.
https://ieeexplore.ieee.org/abstract/document/8395274[4.] Sen, Debashis, and Balasubramanian Raman. "Video skimming: taxonomy and comprehensive survey." ACM Computing Surveys (CSUR) 52, no. 5
(2019): 106.
Egocentric video is captured using body-worn or head-
mounted camera.
The research project: enable video summarization by
detecting and extracting the interactions of the camera
holder with other persons
Method: deep learning model for interpersonal interactions
classification within the video content.
Some challenging cases
:
•
A person is not looking to the camera
•
Video blurring due to ego motion(right images)
•
Persons is out of frame (right images)
Discussion and conclusions
•
Dataset adaptation and preprocess is essential
•
For a feature-based model (was a requirement):
Features require faces. Lack of faces -> no features.
The challenge: how to train the machine to identify the
label “no interaction”? )part of the research)
•
Detection of over 94% of all interactions
•
Generation of interaction-based on personal based vis
summaries.
Video Pre-
processing
Face
Detection
and Tracking
Network
Face recognition:
Unite person’s
IDs
for same video
Model A:
17 Features Per
person, per frame:
•
Temporary IDs
•
Facial Keypoints
•
Bounding Box
•
Detection
Score
•
Frame Number
MTCNN_face_detection_alignment
Lens Correction
Distorted
Egocentric
Video
Undistorted
Video
Image: Per person
per frame
•
Temporary IDs
•
Frame Num
•
image
Model B:
2 Features Per person,
per frame:
•
Face Box area
•
Distance between
eyes
•
Frame Number
face_recognition
Model A: 51 Features
with IDs streamlined
Feature
Streams
Preparation
(for sequence analysis)
Model A: select
features of up to 3
persons per frame
(51 features/ frame)
Model B: select
features of up to 3
persons per frame
(6 features / frame
Temporal
Sequence
Analysis
Network
Always:
•
Frame Number
•
Classification
Probabilities
Options:
1: Per-frame
2. Smoothed
Decision
Generate a
video
summary
Output
Summary
Videos:
•
Summary of
interactions
•
No-interaction
leftovers
•
All frames with
specific ID
Proposed Method 1 – Feature Based Model
CSV with
Decision
per frame